Merck Group’s CVC managing directors Owen Lozman and Hakan Goker speak about the transformational power of machine learning in pharma and materials science.
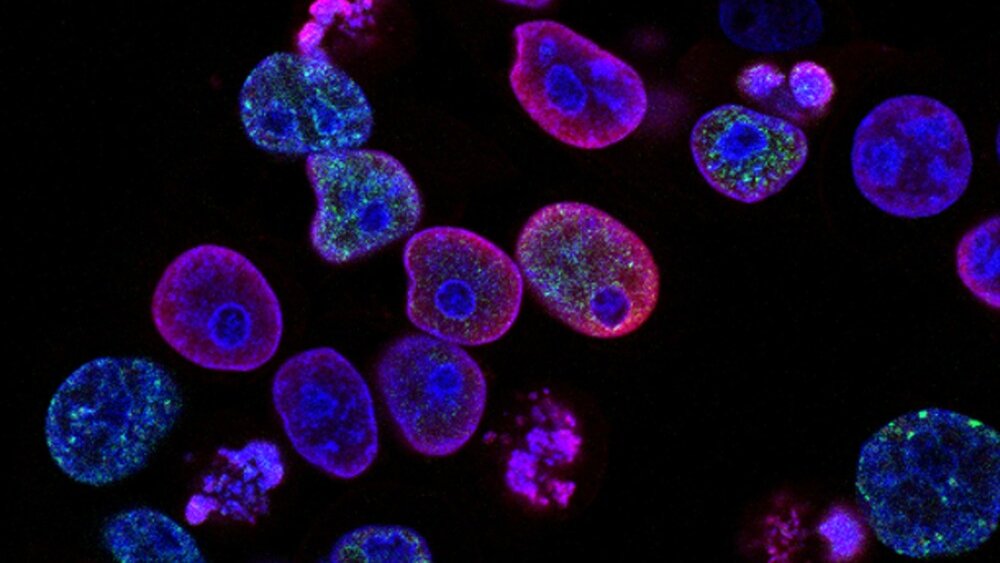
A decade ago, AI was still a vague pipe dream without the computing power to make it an effective reality.
This year has, if nothing else, been the year of AI. No sector of the economy isn’t host to an arms race for the AI product that will get to market first. Every VC outfit with money to spend has been going after it.
The two main sectors where M Ventures, the corporate VC unit of Germany’s Merck Group, is active – biotechnology and new “frontier” technology – are no exceptions.
When the venture market was at its frothy height in late 2021, M Ventures saw its coffers topped up to the tune of €600m to invest over five years, the deployment of which has made for a busy two years so far.
AI
“Over the last five years, we started to invest heavily in things like AI accelerators, knowing that these were going to be technologies which would drive a lot of adoption in the electronics business,” says Owen Lozman, the managing director overseeing frontier and materials technology at M Ventures, on a recent episode of the CVC Unplugged podcast.
“Now it’s really fascinating as we’re seeing these technologies starting to mature and come to the market.”
Much of the technology that the unit has invested in to drive advances in semiconductor materials has been making its way into data centres and other applications where it can, in turn, help healthcare professionals design drugs faster and more effectively.
“The underlying technology is still developing, and really quickly,” says Lozman, highlighting how the sector still needs to iron out much of the detail – securing the data, setting up processes, and ultimately trusting its outputs – before companies can rely on AI.
In healthcare, the applications for AI are legion. Breakthrough-seeking activities like novel drug discovery, where having an engine that can simulate a large number of chemical and biological reactions can speed up the process. In an industry where the drug development process can go into the hundreds of millions – even billions – of dollars, being able to bypass some of the trial and error can save a lot of money not just for the business but for the patient.
“[AI applications] could potentially in the future drive prices of drugs lower because you haven’t just spent that much money on R&D, so you can actually afford to have different pricing on these drugs,” says Hakan Goker, M Ventures’ managing director, who oversees biotechnology.
Everyday functions like immunohistochemistry – common in pathology labs and which typically involves using staining agents to track chemical processes at the cellular level – can now be done digitally with AI, as is the case with M Ventures’ Los Angeles-based digital pathology portfolio company Pictor Labs.
Using data
AI doesn’t operate in a vacuum; it needs to be constantly fed with data to train and refine the models. How that data is collected, processed and protected become a crucial consideration in any context, especially when it comes to patients’ medical data.
“There are obvious concerns because owners of the tools, owners of the processes, don’t necessarily want a software company or a chemical company to have access to all of this data. They want to have the benefit of the model, they want to have the benefit of the insights which it develops, but they don’t want anybody to see the data that’s gone into forming that model,” says Lozman.
One potential route that is already being taken is federated learning, where multiple organisations train a machine learning algorithm without exchanging data themselves. Instead of the usual methods that involve the collation or large amounts of data to train a model from single sessions, it can be broken up into multiple sessions, each with its own siloed dataset, while still being able to collaborate.
There’s a lot of AI which is a little bit less sexy… about trying to take data that exists already and try and get more insight from it
Owen Lozman
Both semiconductors and healthcare have long and costly development cycles. While there are no FDA-type regulators for semiconductors, the customers and chipmakers themselves act as de facto regulators and assess the technology’s effectiveness.
“[In addition to creative generative AI applications] there’s a lot of AI which is a little bit less sexy, if you want to call it that, which is just about trying to take data that exists already and try and get more insight from it,” says Lozman.
But data doesn’t have to be sexy to have large impact, especially in an industry where mistakes can get very expensive. One portfolio company, Tignis, uses AI and machine learning to help chip manufacturers accurately predict how their equipment can be used in different ways – and where they can go wrong – before having to try it. It can streamline and change chipmaking processes in a less risky way.
“You don’t change anything in the semiconductor manufacturing process unless you really have to. You can have huge unintended consequences and cause a lot of cost,” says Lozman.
A future with less disease
Health innovation is solving the most stubborn diseases humanity has known.
There have been cancers, which have been incurable – like childhood diseases, certain leukaemias – which are now 100 percent curable
Hakan Goker
One of M Ventures’ latest investments, Swiss cancer immunotherapy company Nouscom, develops vaccines that can be administered to multiple patients rather than having to be customised to a single patient. Vaccines, more widely, have also seen advances in the speed they come to market, as evidenced by the mRNA vaccines that were produced at breakneck speeds during the pandemic.
“Drug development and disease modification have changed significantly over the last 10 to 15 years. There have been cancers, which have been incurable – like childhood diseases, certain leukaemias – which are now 100 percent curable,” says Goker.
The future looks full of novel drug approaches, with work being done everywhere from academia to corporates to startups. The computational power available today, and the greater capacity for information sharing means less siloing of research and faster collaboration, while the scale of the data available to work with is only expanding as imaging and other technologies advance.
You can listen to the entire conversation on the CVC Unplugged podcast.
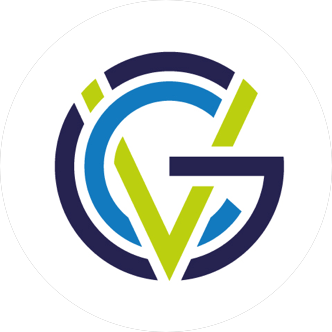
Fernando Moncada Rivera
Fernando Moncada Rivera is a reporter at Global Corporate Venturing and also host of the CVC Unplugged podcast.